The Importance of Bounding Box in Data Annotation and AI Applications
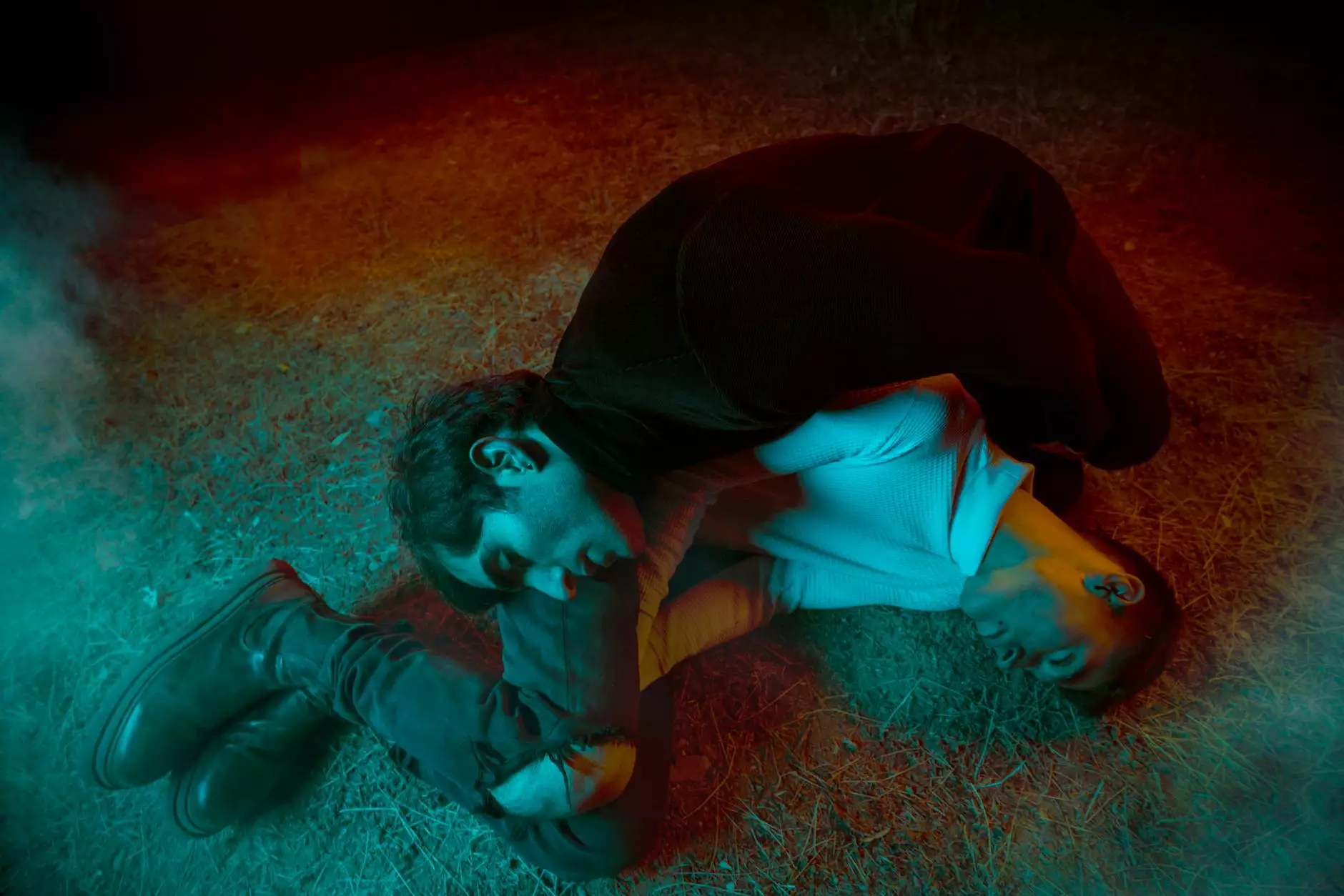
In the era of artificial intelligence (AI) and machine learning (ML), bounding box annotations have become essential tools for developers, researchers, and businesses that utilize computer vision technologies. The term "bounding box" refers to the smallest rectangle that can fully encompass a particular shape or object within a dataset. Understanding the importance and applications of bounding boxes can considerably enhance the quality of AI models and their ability to understand visual data.
What Is a Bounding Box?
A bounding box is a simple yet powerful concept chiefly used in the realms of computer vision and graphics. It provides a method for including every pixel of a target object, regardless of its contour or shape. This technique is vital for tasks such as object detection, image segmentation, and video tracking.
Bounding boxes are generally delineated by four parameters: the coordinates of the top-left corner, the width, and the height of the rectangle. These parameters help define the exact space occupied by the object in an image or video frame.
Applications of Bounding Boxes in Business
Bounding boxes prove valuable across numerous sectors, enabling businesses to leverage AI technologies more effectively. Here are some notable applications:
- Automated Quality Control: In manufacturing, bounding boxes can identify defects in products by isolating areas that do not meet quality standards.
- Retail Inventory Management: Retailers utilize bounding boxes to track and manage stock levels in visual data from surveillance cameras.
- Autonomous Vehicles: Self-driving cars employ bounding boxes to detect and react to various objects, including pedestrians, other vehicles, and traffic signals.
- Healthcare Imaging: In medical imaging, bounding boxes assist in identifying areas of interest within scans, allowing for better disease diagnosis and treatment planning.
How Bounding Box Annotations Improve AI Models
Bounding box annotations hold significant value in training AI models for various applications. Here’s how:
1. Enhanced Object Detection
The use of bounding boxes allows AI models to learn the precise locations of objects within images. By highlighting areas of interest, models can become adept at recognizing and categorizing objects in diverse settings.
2. Better Data Quality
For AI models, data quality is paramount. Accurate bounding box annotations contribute to superior training datasets, enabling models to generalize better and provide more reliable outcomes.
3. Streamlined Workflow in Data Annotation
Using bounding boxes streamlines the data annotation process. Annotation tools that incorporate bounding box functionality can automate part of the labeling process. This leads to faster turnaround times and reduced costs in data preparation.
The Bounding Box Annotation Process
The process of creating a bounding box involves several steps, which include:
1. Image Selection
The first step is selecting images that contain the objects that need annotation. This is a crucial phase since diverse images contribute to the robustness of AI models.
2. Manual or Automated Annotation
Once images are selected, annotations can be performed either manually or automatically. Manual annotation involves human operators drawing rectangles around objects, while automated tools use algorithms to identify and draw bounding boxes.
3. Quality Assurance
After annotation, it is critical to perform quality assurance checks to ensure that bounding boxes correctly encompass the objects they describe. This is often done through a review process to catch errors and discrepancies.
Keylabs.ai: Leading the Charge in Data Annotation Tools
At Keylabs.ai, we recognize the pivotal role of bounding boxes in data annotation. Our cutting-edge data annotation tools and data annotation platform are designed to facilitate precise and efficient bounding box annotations. Here are some key features:
- User-Friendly Interface: Our platform offers a straightforward interface that enables users to create bounding boxes easily, whether manually or with the help of automation.
- Collaboration Tools: Teams can work together on projects, ensuring consistency and accuracy in annotations.
- Quality Control Mechanisms: Built-in quality checks ensure that every annotation meets industry standards.
- Customizable Workflows: Users can tailor workflows to fit their specific project requirements, enhancing productivity.
Conclusion
In conclusion, the bounding box plays an indispensable role in the data annotation process, particularly in enhancing AI and machine learning applications. By offering a structured method to identify and delineate objects, bounding boxes significantly improve data quality and model accuracy. As businesses increasingly adopt AI technologies, leveraging effective data annotation practices through tools like those offered by Keylabs.ai can lead to impactful outcomes and competitive advantages.
Embracing bounding box annotation not only accelerates the development of intelligent systems but also creates new opportunities for innovation across industries. We invite you to explore our advanced solutions and enhance your AI initiatives with the power of precise data annotation.