Unleashing the Power of Annotated Image Datasets for Business Insights
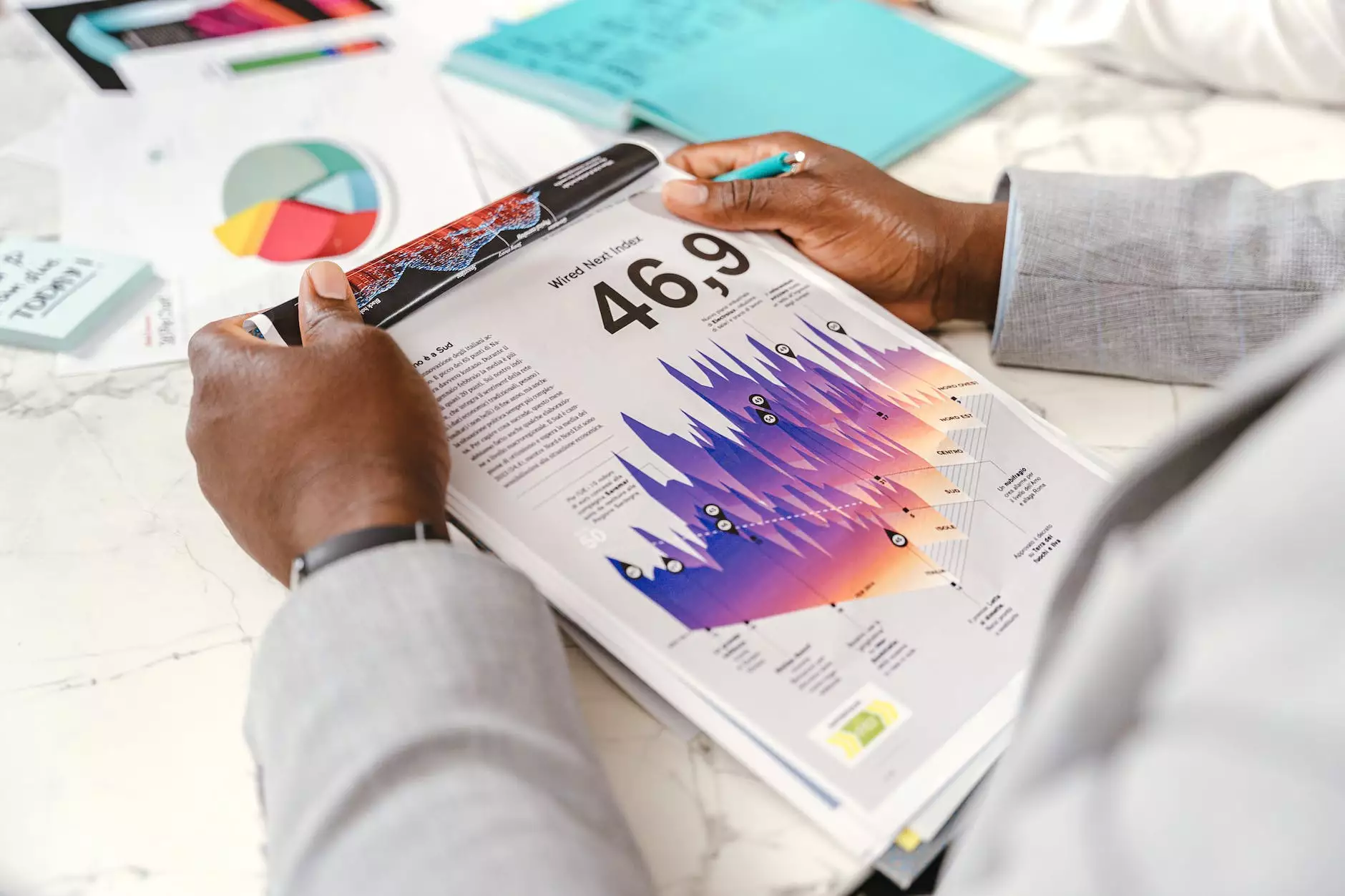
The world of business is rapidly evolving, driven by technological advancements and the increasing importance of data analytics. One of the game-changing components of this data-driven landscape is the annotated image dataset. In this article, we will explore how businesses can leverage annotated image datasets, the utility of data annotation tools and platforms, and the myriad of opportunities presented by this technology. By the end of this comprehensive guide, you will understand how to effectively utilize these datasets to gain a competitive edge.
Understanding Annotated Image Datasets
Annotated image datasets refer to collections of images that have been labeled or tagged with descriptive information. This process occurs through various data annotation techniques that enhance the value of raw image data, making it ready for analysis and algorithm training. The primary objective is to identify and label specific elements within the images, which can later be utilized for various applications, including machine learning, computer vision, and beyond.
The Importance of Data Annotation
The impact of data annotation on the quality of machine learning outcomes cannot be overstated. Here’s why it’s a critical aspect of any data-centric business strategy:
- Quality Training Data: Machine learning models are only as good as the data they're trained on. High-quality annotated datasets improve model accuracy significantly.
- Enhanced Decision Making: By utilizing annotated image datasets, businesses can derive actionable insights that lead to informed decision-making.
- Cost-Effective Solutions: Automating the annotation process can reduce manual labor costs while speeding up project timelines.
- Scalability: Annotated datasets allow for the scalability of machine learning projects, making it easier to handle large volumes of data.
How Businesses Use Annotated Image Datasets
Companies across various industries utilize annotated image datasets in multiple ways:
1. Retail and E-commerce
In the retail sector, annotated image datasets play a pivotal role in enhancing customer shopping experiences. For instance:
- Visual Search: Users can search for products by uploading images, leading to a faster and more intuitive shopping experience.
- Inventory Management: Automated systems can monitor inventory levels by analyzing images of stock shelves.
- Personalized Marketing: By analyzing customer preferences through their interactions with images, businesses can tailor marketing strategies effectively.
2. Healthcare and Medical Imaging
In healthcare, annotated image datasets are invaluable:
- Diagnostic Assistance: Machine learning algorithms trained on annotated medical images can assist doctors in diagnosing diseases more accurately.
- Research and Development: Annotated datasets aid in clinical research by providing the necessary data for analysis and validation.
3. Autonomous Vehicles
In the automotive industry, especially in the development of autonomous vehicles:
- Object Detection: Annotated datasets enable vehicles to recognize and react to various objects on the road.
- Road Condition Analysis: By training algorithms on annotated images of road conditions, vehicles can adapt to different terrains and climates.
Creating an Annotated Image Dataset
Building an annotated image dataset requires a systematic approach:
Step 1: Define Objectives
Before you start, clearly define the objectives behind creating the dataset. Understanding the end goal will guide the annotation process.
Step 2: Data Collection
Gather images that fit your objectives. Sources can include stock photo repositories, user-generated content, or custom photography.
Step 3: Choosing Annotation Tools
Select appropriate data annotation tools or platforms to facilitate the process. Some popular platforms include:
- Keylabs.ai - A robust data annotation platform offering a variety of functionalities.
- Labelbox - A comprehensive solution with collaboration features.
- RectLabel - A handy tool for annotating images for machine learning.
Step 4: Annotation Process
Engage skilled annotators to label the images accurately. The quality of annotations impacts the effectiveness of the dataset.
Step 5: Quality Assurance
Implement a quality assurance process to review and correct annotations. This will ensure high-quality outputs that can produce valuable insights.
Data Annotation Tools and Platforms
With the rise of the need for annotated image datasets, various tools and platforms have emerged to simplify the annotation process:
Keylabs.ai
Keylabs.ai offers a state-of-the-art data annotation tool designed to streamline the image annotation process. Its features include:
- Automated Annotation: Use AI-assisted tools to speed up the labeling process.
- Collaboration: Teams can work together seamlessly, ensuring efficient workflows.
- Flexibility: Customize the annotation workflow to suit specific project needs.
Other Notable Platforms
Besides Keylabs.ai, other platforms offer additional features that might suit various business needs:
- SuperAnnotate: Ideal for projects requiring high-quality annotations with multiple formats.
- Scale AI: Focuses on providing an array of data services alongside annotation.
- Snorkel: A unique platform that leverages weak supervision to automate annotation.
Challenges of Data Annotation
While the benefits of using annotated image datasets are profound, businesses may encounter challenges during the annotation process:
1. Labor Intensive
Manual annotation can be time-consuming and requires significant human resources.
2. Subjectivity in Labels
Different annotators may interpret tasks differently, leading to inconsistencies within the dataset.
3. Machine Learning Bias
Biases in datasets can lead to unfair or inaccurate machine learning models. Ensuring diversity in your training data is vital.
The Future of Annotated Image Datasets in Business
The future of annotated image datasets looks promising as industries increasingly rely on data-driven decisions. Here are some trends to watch:
1. Increased Automation
As AI technologies advance, expect more automated data annotation processes, reducing reliance on manual labor.
2. Enhanced Collaboration
With remote work becoming more prevalent, platforms will focus on facilitating collaboration among global teams.
3. Focus on Ethical Data Usage
Awareness regarding ethical data practices will push businesses to adopt fair data annotation methodologies, promoting diversity and reducing bias.
Conclusion
In conclusion, embracing annotated image datasets offers a wealth of opportunities for businesses eager to enhance their data strategies. The ability to gain insights, improve decision-making, and drive innovation is crucial in today’s competitive landscape. By utilizing effective data annotation tools and platforms like Keylabs.ai, companies can unlock the full potential of their data, leading to robust growth and success. As the data landscape evolves, prioritizing quality annotation practices will be essential for harnessing true business value.
Investing in data annotation not only prepares businesses for future challenges but also positions them as leaders in their respective markets. The time to leverage annotated image datasets is now, and the possibilities are limited only by your imagination.